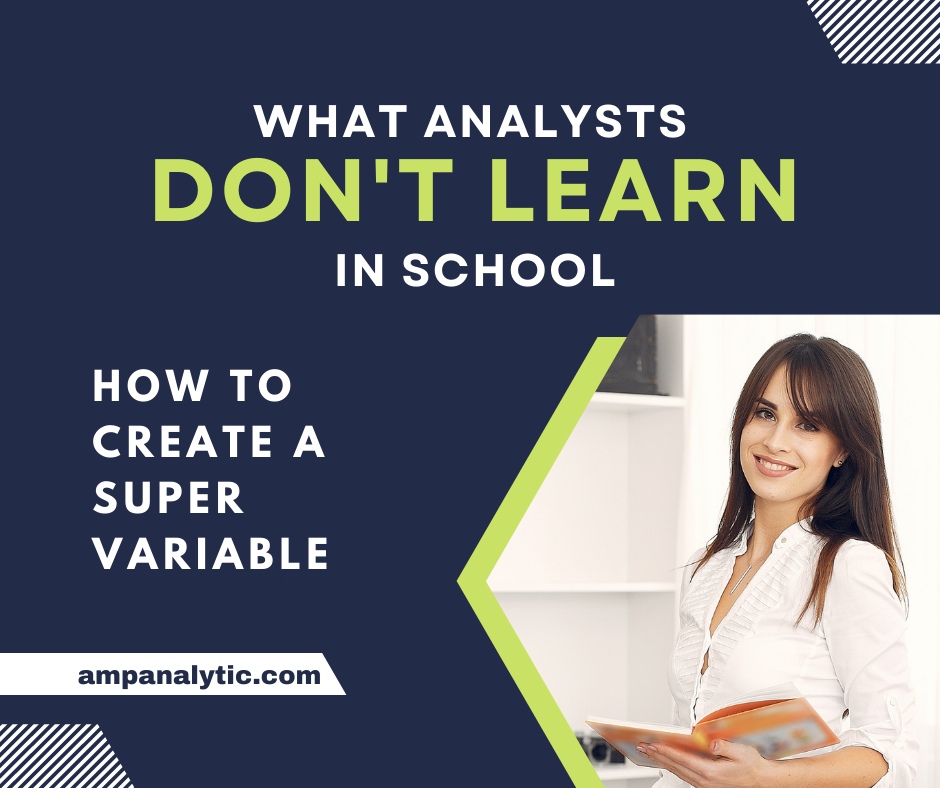
In statistics class, students learn how to calculate values and to build statistical models. What they don’t teach you in class is how to transpose variables into extremely meaningful insights. If you want to take mediocre data and build out awesome client solutions, you need to learn to be creative. Data points by themselves may seem weak and limp. If you learn the data science strategy of combining, dividing, multiplying and factoring, you can truly change the statistical laws of gravity.
Create a Super Variable
Here is a simple example of taking two spatial data variables to create a super variable solution. In this case, we are considering our GeoGrid spatial data variables of Population and Home Value. In other words, what is the average population within GeoGrids and what is the average Home Value within GeoGrids. For definition, GeoGrids are our perfect squares of spatial data. They can be as large as 1/4th of a square mile but as small as 1/16th of a square mile depending on the density of the population. These two variables by themselves seem weak as statistical predictors, but when we divide one into the other, that’s where the magic happens.
In this example, Population density has a variable power (predictive strength of the variable) of 1.55 and Home Value has a variable power of 1.20. However, when we divide Population by Home Value the newly constructed values have a variable power of 4.55.

What Power Does Your Existing Data Have?
This is a single-factored variable and will ultimately be only one of 10 to 15 variables that make it into the final model. Just think if your final model had 5 variables with this kind of power included in it. Many times your existing data set may already have tremendous power just waiting to be tapped into. It is just a matter of developing a strategy for extracting truly meaningful value from what you already have in place.
At AMP, we have some truly unique variables, and we want to help analysts take a fresh look at the data you are already using to seek out and learn what is possible with data transposition. The same types of impact are seen with transactional data, household level demographics, and spatial data alike.
If you are not already creating custom data variables, try it out. We would love to hear how this idea is changing your internal view of analytics. Share your story with us at amplify@ampanalytic.com.
If we can assist you further in your marketing analytics endeavors, please let us know.
Written by Steve Godwin, CEO Analytic Marketing Partners